Computer vision is becoming increasingly integrated into our lives. At the same time, we do not even notice all this observation. Today we will talk about a system that helps to analyze the emotions of visitors at conferences, in the educational process, in cinemas and in many other places. By the way, we will show the code and tell you about practical cases. Look under the cat!
I give the word to the author.Want to always know in person the audience in the hall, classroom, office and manage it? The question is not idle. This is an offline audience. To improve business performance, having information about your client's behavior, reactions and desires is vital. How to collect these statistics?
With an online audience, everything is easier. If the business is on the Internet, then marketing is greatly simplified and collecting data on the profile of its customers, and then also “catching up” it indefinitely - simply. A set of tools - take it and use it, the cost of lifting.
In offline mode, the same methods are used as 50 years ago: questionnaires, questionnaires, external observers with a notebook, etc. The quality of these approaches leaves much to be desired. Reliability is doubtful. About convenience in general can be silent.
In this article we want to talk about the new solution of the company CVizi, which collects interesting and unique statistics and helps to analyze information about your customers / visitors using computer vision.
Video analytics and cinemas
Initially, the product was developed as a tool for counting the audience of the cinema and comparing their number with the purchased tickets for disciplinary control of staff and the prevention of violations. But as they say, the appetite comes with eating.
The developed technology has expanded the capabilities of the solution. It became possible to determine the age and gender characteristics of cinema visitors, as well as to determine the emotional background of each person during the session.
Information is updated promptly and the user only needs to log into the personal account and view statistics on the session of interest or an analytical report on the audience.
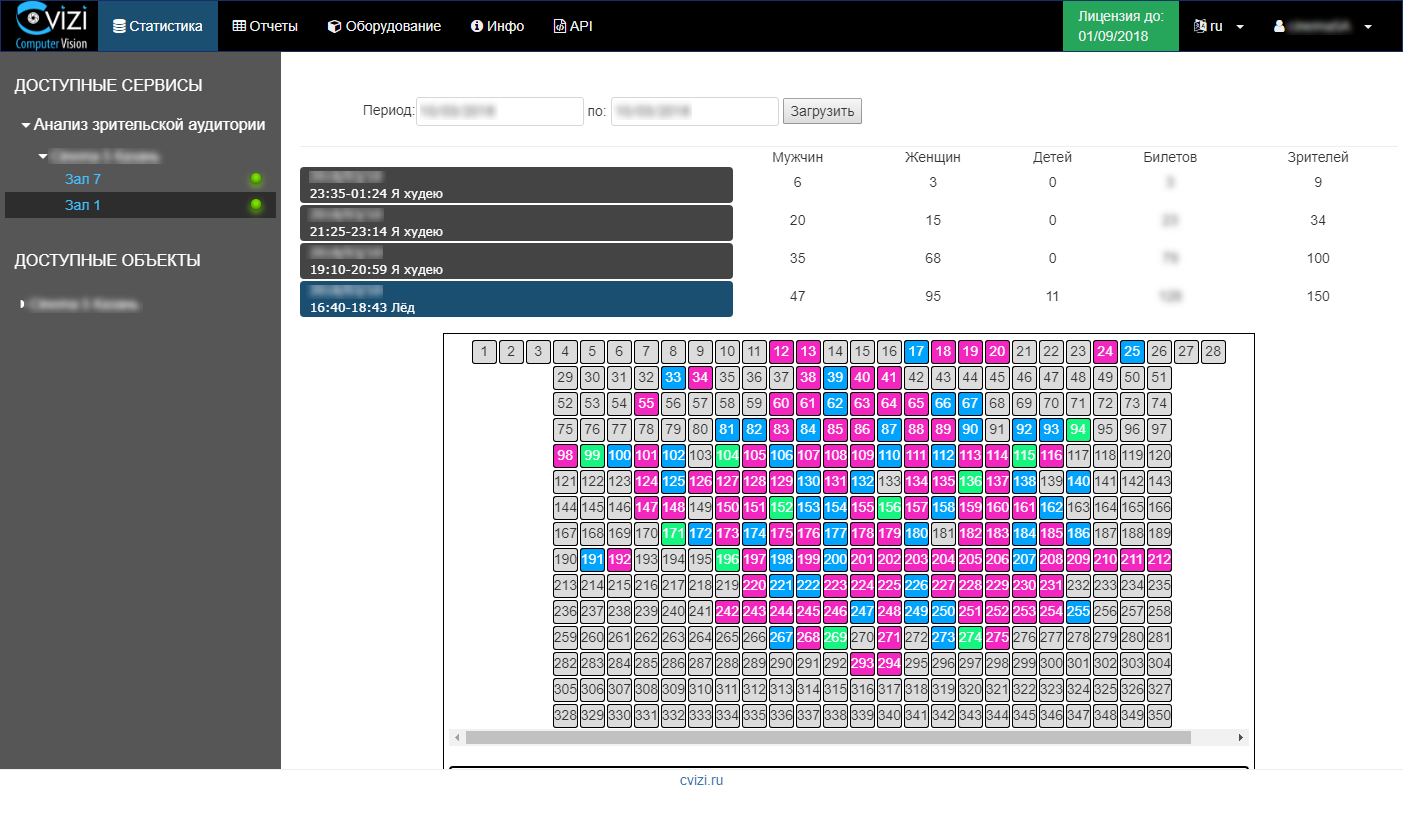
For example, we open a session with the film “Ice” and see on the realogram of the hall a clear dominance of the female audience (in principle, predictable). And then look at the emotions that people experienced (you can see each).
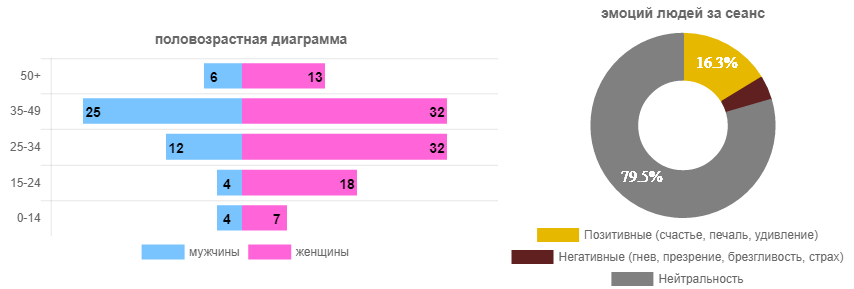
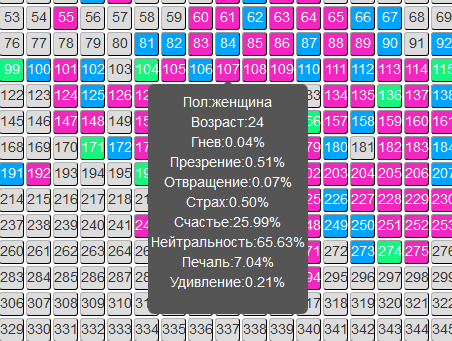
This was the data for one specific session. Now let's see the same data as a whole for the entire movie rental period (in one cinema) and get a more relevant picture.
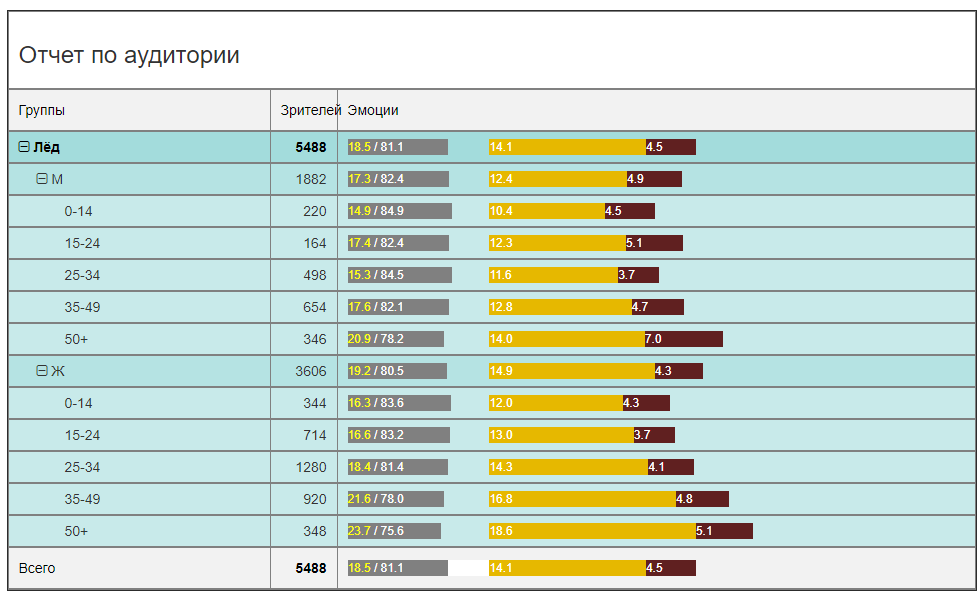
In terms of attendance, there is a clear domination of female viewers aged 25-34 years. There are 2.5-3 times fewer men. Those. put in the ad unit advertising a new SUV is not as reasonable as a new mascara. Although this is a moot point. But in any case, after a couple of days of rental, you can safely guarantee the advertiser target audience, and even in quantitative terms.
From the point of view of the emotional background, the picture will be interesting first of all for distributors and distributors. Who is the film happy and annoying? Should I continue to shoot? Believe bots reviews or get reliable statistics? In this case (the film “Ice”) the film is quite bright in terms of emotions. The amount of positive and negative emotions is 18.5 parrots out of 100. The rest is neutral. This is quite a good indicator. For comparison, the emotional background in other films may not be so rosy (on average in the hospital for all ages and genders).
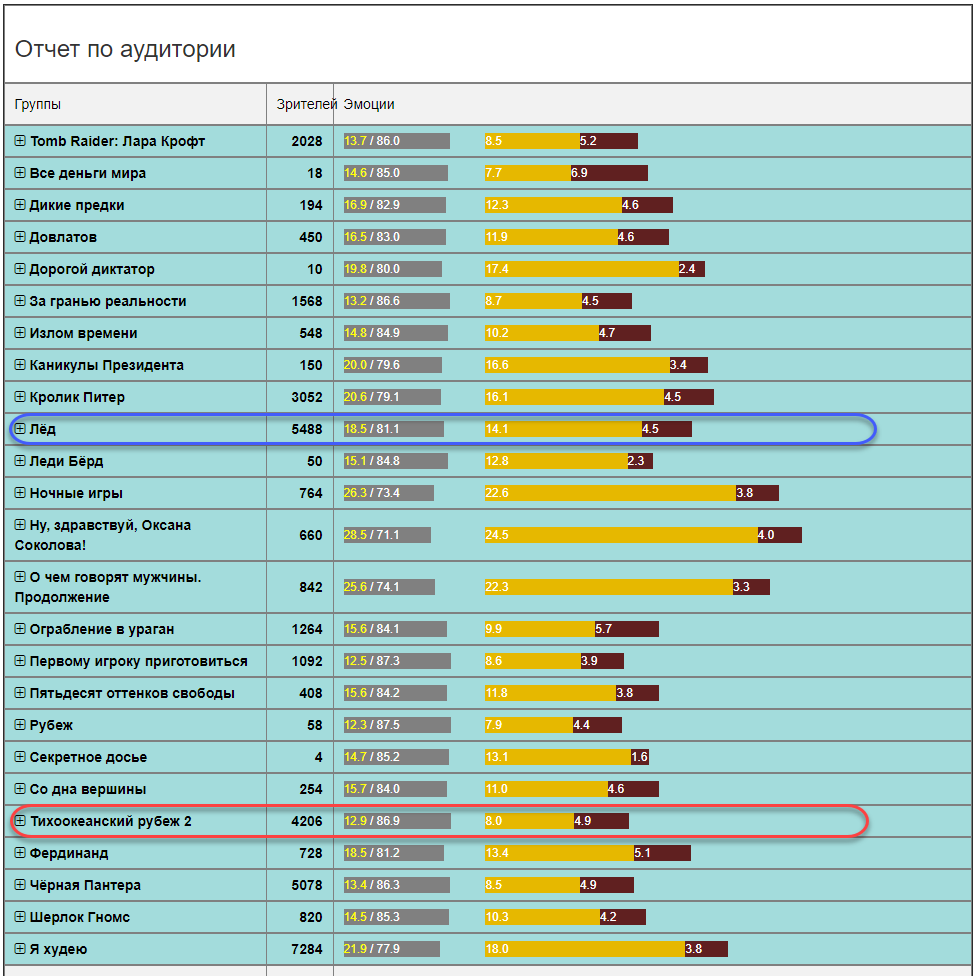
For example, the “Pacific Line” with a comparable number of viewers over the same period has positive emotions 2 times less. And the film “Well, hello, Oksana Sokolova” is a simple discovery for women “50+”.
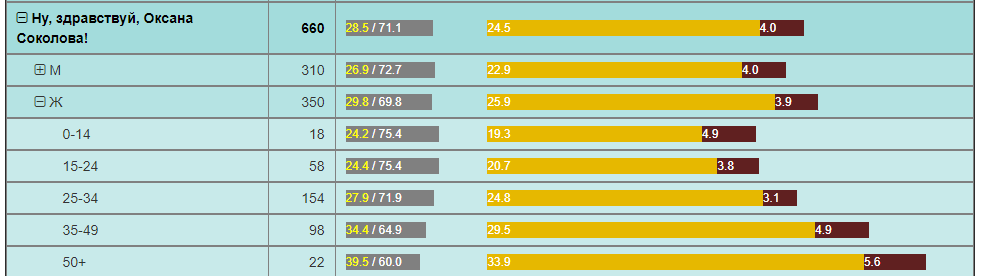
All of these are some examples of analysis that can be used in movie networks. They simply did not have this information and very few people know that it can be obtained at all, much less used for their own purposes.
For cinema chains, there are great opportunities for a deeper analysis of what is happening in the hall according to the composition of the audience and their reaction to films in various localities.
You can immediately identify the following :
- The ability to test any marketing hypotheses - to receive feedback in real time and objectively assess the change in the audience and the effect of marketing activities.
- The ability to provide advertisers with accurate information about the audience that viewed the ad. Moreover, it is possible to guarantee the advertiser viewing of the advertisement by certain viewing groups.
- The ability to create unique marketing offers (discounts) on the market for visitors of a certain age and gender, without breaking the existing scheme of work and without reducing the current financial indicators.
These and many other opportunities that have emerged due to this decision can turn the cinema network market upside down, increase revenues and improve the operating efficiency of both individual cinemas and the network as a whole.
Given that the solution is “easy” (no servers, no stream to the Internet), actively uses the cloud from Microsoft Azure and has affordable prices for the market (in the form of periodic subscriptions to the service), cinema networks in Russia and abroad are actively implementing it.
Moreover, the created technology is reflected in other solutions in parallel areas.
Video analytics and events
Events, events, forums, summits, conferences, symposia ... What do the organizers know about the audience that came? X people were registered, Y came, Z filled out the questionnaire. That's all.
Questioning is a separate pain: “So, I know this comrade well, I will give him 5 points, although the report was tedious.” We lead to the fact that the reliability of the questionnaires is low.
About understanding the effectiveness of the conference, feedback from participants to the organizer and sponsors is essential.
The audience analysis service in cinemas is perfectly transferred to the conference venue. CVizi also tested this case by conducting two experiments: in the Matrex hall in Skolkovo at one of the conferences and in the Digital October hall during the Russian finals of the student technology projects competition Imagine Cup 2018.
Experiment 1. Matrex Hall in Skolkovo
In Matrex, the camera was installed not above the screen, like in a movie theater, but on a telescopic stand behind the speakers. Thus, the solution was mobile. It takes only a few hours before starting to install a camera, set up a planogram of the hall and enter the schedule of speakers' speeches. Then the system does everything itself.

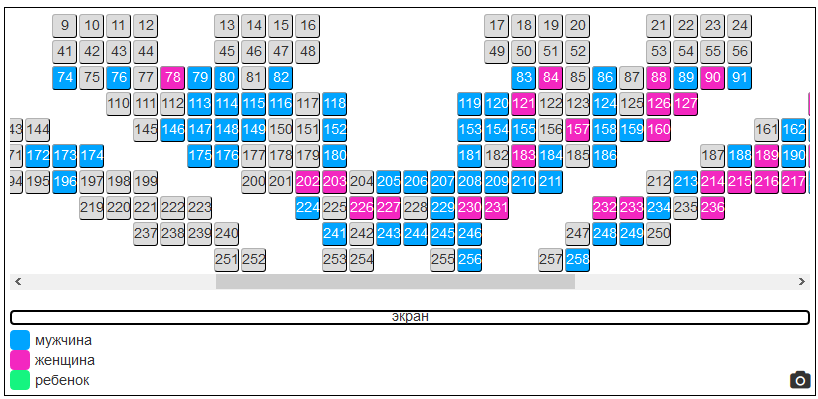
Now the organizer can collect data on the quality of reports through the number of participants in the hall and their emotions at each session / report. And the next time you can plan an event, based on objective facts. There are reports, after which the participants simply leave the hall, and the next speaker works with those who stayed or did not have time to leave. This is wrong, but such a forecast could simply change the effect by simply rearranging the reports.
Experiment 2. Russian final of the Imagine Cup
Here the goal was somewhat different, but the approach is the same. One of the contest nominations is the Audience Award. It is those viewers who sit in the hall, not online viewers. And for the first time in the Imagine Cup, the team chosen by artificial intelligence won the audience award. The team won, to whose performance the audience reacted most positively while the guys were on the stage. For this, two cameras have already been used, each of which continuously monitored its sector of the hall and collected the emotional characteristics of each viewer.
In this case, the camera was able to hang on the mast of lighting above the stage, which ensured their guaranteed stability and immobility. And also once again made sure that 4G-Internet is enough for the service to work. The system turned out as autonomous as possible. From the organizers it took only food 220 V. This is really important, because at events of this scale, communication channels are always a bottleneck, and the quality of the service’s work directly depends on the stability of the Internet channel.
As a result, the team won the audience award, gaining the maximum number of positive emotions for their performance - Last Day Development from UNN them. Lobachevsky.

Video analytics and educational process
The field of education in terms of automating its processes is still very far behind other industries. For example, in many universities, attendance control is still practiced in the form of an external controller with a magazine: a man came in, counted students at lectures, entered some figure in a magazine (what question?), And went on.
The technology of analyzing the audience using computer vision methods is also excellent for the field of education and opens up a large field for experiment and analysis. Possible solvable tasks:
- Attendance control
- Student identification
- Evaluation of teaching quality
- Identify the student's negative emotions and take preventive measures.
- Assessment of the involvement of the student or student in school.
Proper planning of the educational process, including the schedule, the choice of lecturers is the basis for the success of education. And in fact, if translated into the economic field - is the cost of education in the context of each student.
Video analytics in other industries
CVizi technologies are not limited to the solutions presented, there are solutions for retail and manufacturing.
For example, an interesting solution is to obtain information about the external and internal conversion of the store. These two indicators allow you to build a funnel offline sales:
- How many people pass by your store;
- How many people went into it;
- How many people reached the ticket office.
Here is an example of external conversion for a typical store located in a shopping center in Moscow:
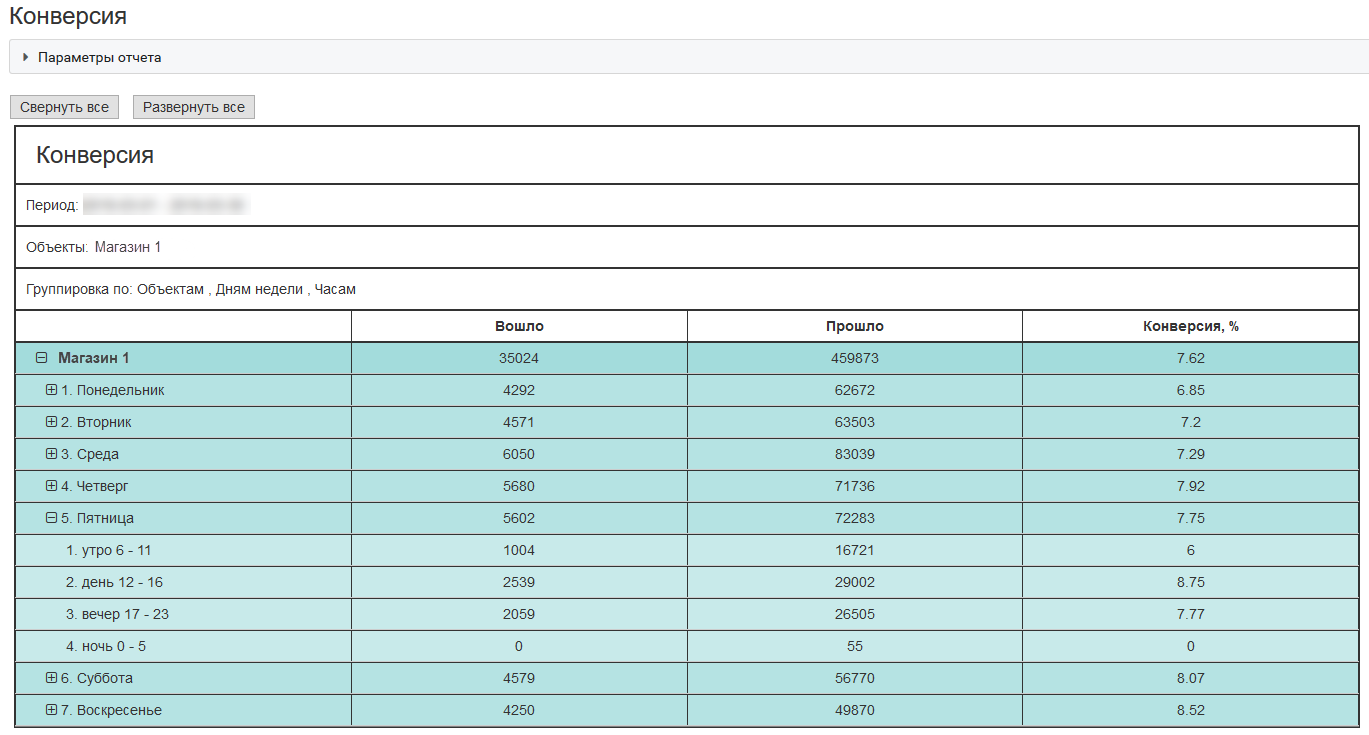
Having these indicators, a business can more efficiently spend money on attracting customers, on testing marketing hypotheses, on quality of service.
How it works?
Now I want to talk a little about technology. Why do we say the solution is “easy”? It's all about architecture. Any customer is always in danger of buying an expensive solution and in addition to him a fleet of vehicles in the form of various servers, registrars and other things. And then all this is set up, mated with each other and accompany. If the customer is offered a box in the form of a microcomputer, the size of a mobile phone, then any person, at least a little familiar with the equipment, can install it into his local network and hang an IP video camera. It begs the analogy with a Wi-Fi router at home - plugged into a power outlet, connected the Internet, made the simplest settings. Everything. No service is needed to start. Everything is very easy to do yourself.
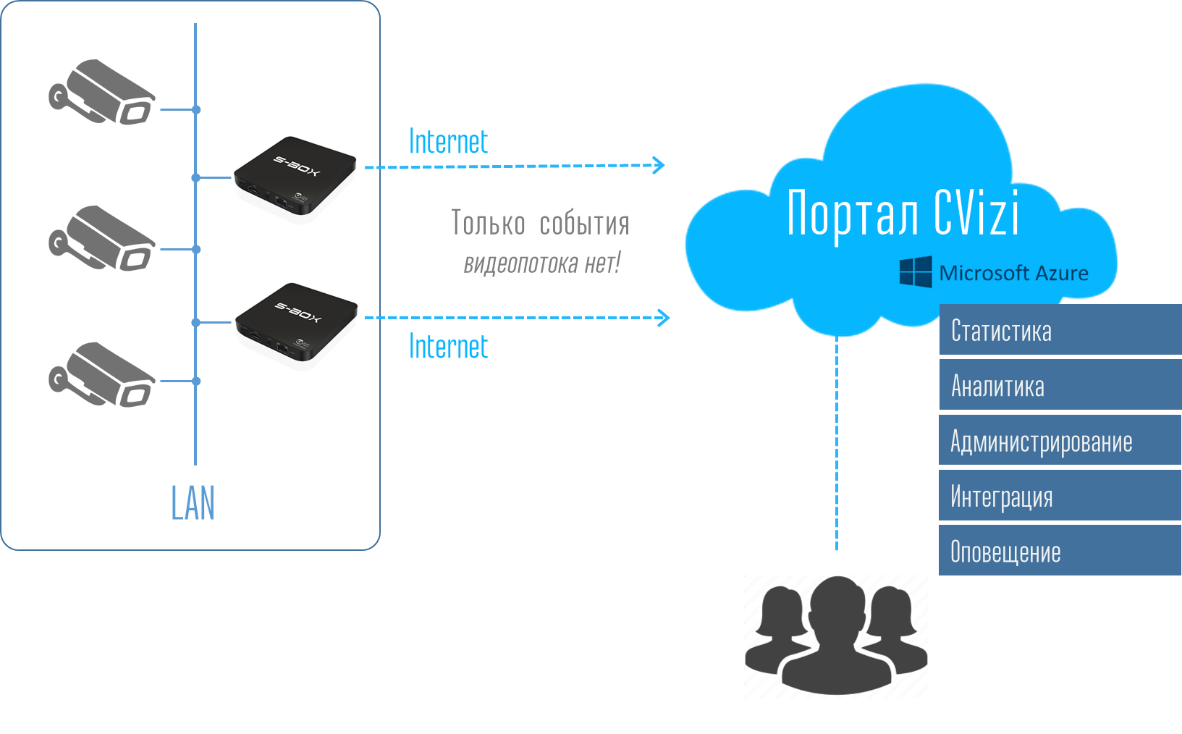
And then in the personal account, the user sees the entire system as a whole and can receive data in the form of reports and statistics journals, and, using the API, take this data into its corporate IT system.
Thus, the user does not need to think and care about the infrastructure. Connecting S-Box computing devices is easy. It is also easy to replicate the solution to other points - hung up the camera, connected the required number of S-Box and immediately see the object on the portal in your account.
Under the portal and personal account there is a whole system of analytics, database repositories, equipment monitoring and notifications, deployed in Azure clouds. The system is elastic and all necessary computing resources are allocated to the user automatically. Therefore, all the headache on the equipment and its maintenance from the user is removed. He buys only a video data analysis service. Very comfortably.
What cameras can be used for video analysis of the audience?
Given that in the end you need to get the viewer's face in acceptable quality, the camera should be, firstly, with good optics and zoom, and, secondly, controlled - PTZ.
We tested more than a dozen cameras from different brands. We will not name specific camera models, but you can voice the characteristics.
For halls up to 150-200 people, camera 20x with a 2Mp matrix is sufficient. For halls of more than 200 people it is better to use cameras 25x and higher. They are undoubtedly more expensive, but this is the right way. Although, of course, the size of the matrix can also be played, but it should be understood that in the IR spectrum the matrix will make noise and the optical zoom will always be preferable to digital.
Recognition accuracy
The closer we position ourselves on the face, the clearer it will be. It seems that everything is clear, and the child is clear. But if with gender recognition everything is fine, then the accuracy of determining the age can easily be ± 5 years and even more, regardless of the approach of the camera. And it's not about the quality of algorithms and neural networks, but the fact that every adult person tries to look as much as he invested in his appearance.
Therefore, the camera will give the age at which a person looks or tries to look. Those. biological age and the age of the photo - it can be two big differences. After all, each of us can remember the cases when he was mistaken in the age estimate of the interlocutor. At the same time, the human brain is a very powerful and well-trained neural network.
Azure and its services
The heart of all this is the Microsoft Face API cognitive service. This is a very convenient service and the API can be accessed from almost any common development environment. We use most of our services using Python - this is on the cloud side.
The overall sequence is as follows:1) S-Box transmits images to the cloud
2) The cloud service accumulates them, sorts and feeds them to Face detection Cognitive Services. For shipment of collected images to the cloud, use Python:
def upload_file(file_path, upload_file_type):
""" Uploading file """
...
if authData == '' or authData == 'undefined':
...
try:
r = requests.post(param_web_service_url_auth, headers={'authentication': base64string})
if r.status_code == 200:
authData = json.loads(r.text)['token']
result = upload_file(file_path, upload_file_type)
return result
logging.error("Upload_file(%s) - UNEXPECTED AUTH RESULT CODE %d", file_path, r.status_code)
except Exception as e:
logging.error('Upload_file(%s) error', file_path)
logging.exception('Upload exception ' + str(e))
return False
try:
base_name = os.path.basename(file_path)
txt_part, file_extension = os.path.splitext(base_name)
files = {'file': open(file_path, 'rb')}
...
if upload_file_type == 1: # for upload full view
...
payload = {'camid': cam_id, 'dt': dt, 'mac': mac}
z = requests.post(uploaddatalinkhall, headers={'authentication': authData}, files=files, data=payload)
if z.status_code == 200:
return True
logging.debug('post result -> %d', int(repr(z.status_code)))
if upload_file_type == 0:
...
payload = {'camid': cam_id, 'dt': dt, 'json_str': json_str, 'mac': mac}
...
z = requests.post(param_web_service_url, headers={'authentication': authData}, files=files, data=payload)
myfile.close()
if z.status_code == 200:
...
if len(debug_path) > 0:
# move
fnn = debug_path + os.sep + json_file
shutil.move(upload_folder + os.sep + json_file, fnn)
else:
# delete
os.remove(upload_folder + os.sep + json_file)
return True
result = "UNEXPECTED STATUS CODE " + repr(z.status_code)
logging.error('upload_file(%s) - ERROR. Res=%s, text: %s',
json_file, result, z.text)
...
except Exception, e:
...
logging.exception('Upload exception: ' + str(e))
return False
3) Python
CF.face.detect , , MS Face detection Cognitive Service API, :
def faceapi_face_detect(url):
…
# API call
image_url = url
need_face_id = False
need_landmarks = False
attributes = 'age,gender,smile,facialHair,headPose,glasses,emotion,hair,makeup,accessory,occlusion,blur,exposure,noise'
watcher = elapser_mod.Elapser()
try:
api_res = CF.face.detect(image_url, need_face_id, need_landmarks, attributes)
# callback(url, need_face_id, need_landmarks, attributes, e.elapsed(), api_res)
text = 'CF.face.detect image_url {}, need_face_id {}, need_landmarks {}, attributes {}, completed in {} s.'. \
format(image_url, need_face_id, need_landmarks, attributes, watcher.elapsed())
printlog(text)
text2 = 'Detected {} faces.'.format(len(api_res))
printlog(text2)
text3 = '{}'.format(api_res)
printlog(text3)
except CF.CognitiveFaceException as exp:
text = '[Error] CF.face.detect image_url {}, need_face_id {}, need_landmarks {}, attributes {}, failed in {} s.'. \
format(image_url, need_face_id, need_landmarks, attributes, watcher.elapsed())
printlog(text)
text2 = 'Code: {}, Message: {}'.format(exp.code, exp.msg)
printlog(text2)
message = exp.msg
…
return api_res, message
. () . . , . . – .
? ! . API. – , , . , () .., «» . . .
.
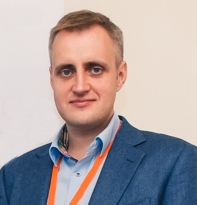
— CVizi. : 'aosipov @ cvizi.com'.
FB .